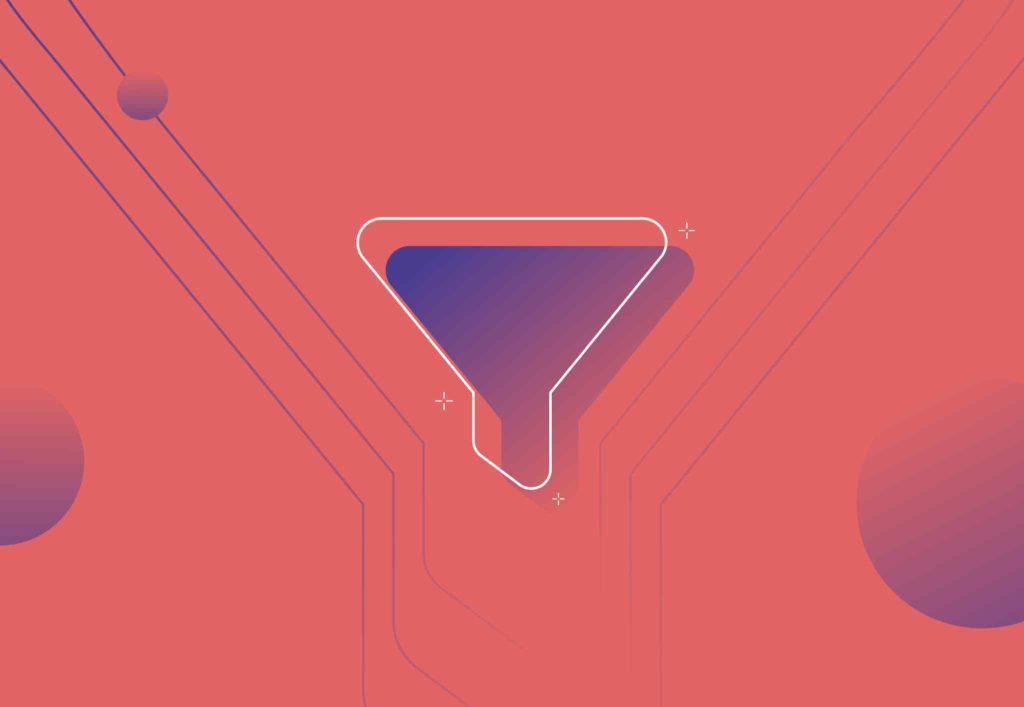
What are recommendation algorithms?
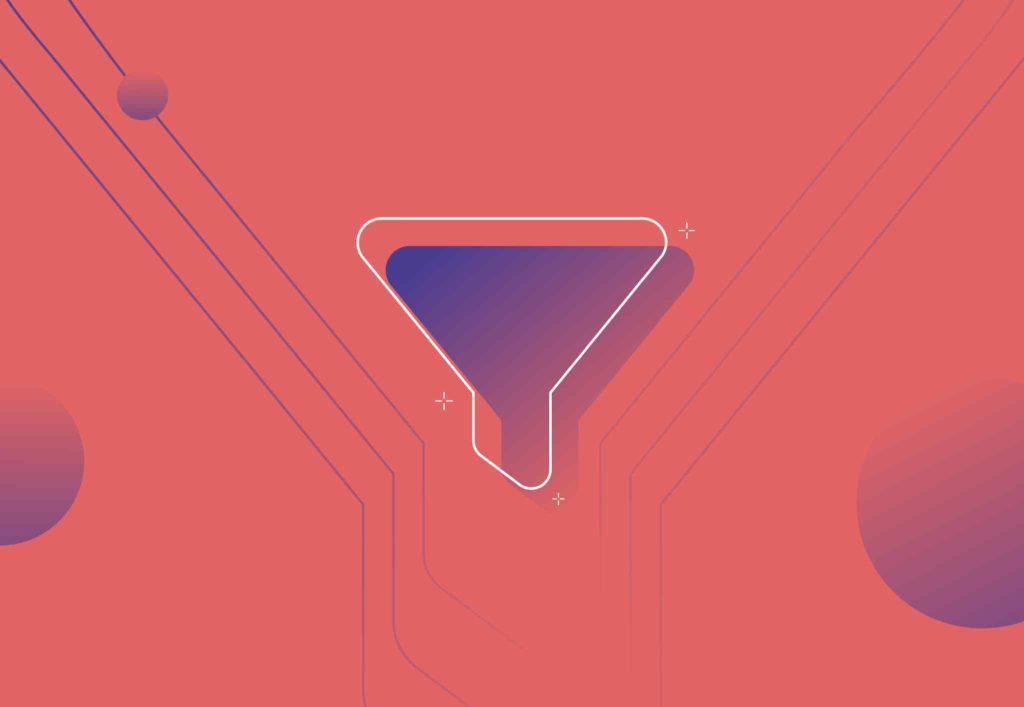
Recommendation algorithms have become increasingly popular in today’s digital and paid social media world, from personalised music playlists, product recommendations, Netflix suggestions and even the content you are shown on social media platforms. These recommendation algorithms are designed to provide personalised recommendations based on user behaviour, preferences, and previous interactions and engagements. They are sometimes referred to as content-first algorithms and have been attributed to the success of TikTok and its most popular influencers.
It is fair to say that this new way of delivering content to users has completely transformed the social media landscape. Since the arrival of TikTok and its algorithm, which has made famous people go viral over pieces of content without having to rely on already having thousands of millions of followers, other companies such as Meta and Twitter have introduced Reels and For You pages in order to retain users who may have left for the Chinese company. This alone is proof that recommendation algorithms are here to stay.
Here at Embryo, our paid social team are well aware of the rise of these content-first algorithms and the need to create content that satisfies them. While growing an online following is important, today, content comes first. Get that right, and the following and brand awareness will follow. If you want to learn more about paid social and how our campaigns keep up to date with the latest updates to the major platforms to ensure results then get in touch today.
So, what exactly is a recommendation algorithm?
A recommendation algorithm is a combination of a dataset and machine learning that provides personalised recommendations based on users’ historical data. The recommended algorithm is used in recommender systems as is a type of data filtering that helps to predict user preferences and interests, making it easier to find content or products they might like or be interested in. Rather than showing content you’ve chosen to see, such as the pictures and posts of people you follow, these kinds of algorithms show content they think you’ll be interested in based on previous interactions.
How do recommendation algorithms work?
Most algorithmic recommendations are calculated using the following steps to provide relevant items and resources to individual users based on their previous interactions.
- Step 1 – Collection
- Step 2 – Storage
- Step 3 – Analysis
- Step 4 – Filtering
Since recommendation algorithms use a combination of data and machine learning, these recommender systems are becoming a lot more common on platforms we use in everyday life and are starting to use a variety of different methods to offer recommendations.
Collaborative Filtering
Collaborative filtering is a type of recommendation algorithm used in recommender systems that recommends items to users based on the preferences of similar users. The premise behind collaborative filtering is that people who have similar preferences or behaviours are likely to have similar opinions on items they have not yet interacted with. This type of algorithm first builds a model of the user-item interactions or ratings, then it looks for users with similar behaviour patterns and recommends items that those similar users have shown an interest in but that the target user has not yet seen or interacted with.
Content-Based Filtering
Content-based filtering is a type of recommendation algorithm used in recommender systems, which works by recommending items that are similar to the items that a user has shown interest in, based on the content or attributes of those items. The algorithm creates a profile of the user’s preferences based on their interactions with those items, then looks for items with similar content or attributes to those previously interacted with and recommends them to the user. For example, if a user has shown a preference for action movies, the algorithm may recommend other action movies with similar themes, characters, or settings.
Hybrid Recommendation Systems
A hybrid recommendation system combines both collaborative filtering and content-based filtering to provide more accurate and diverse recommendations which help it overcome the limitations of individual methods and improve the overall performance of the recommender system.
User-User Algorithms
A user-user algorithm recommends goods and services that other people who are similar to that user would view. It views the number of items they have in common based on an ever-growing data set. An algorithm may deem two users similar if they engage with relevant content on a particular social media platform or follow the same people.
User-item algorithm
Often used in recommender systems, a user-item algorithm suggests items to groups of users based on prior behaviour or preferences. It analyses individual user behaviour, looking at things such as clicks, purchases, and product ratings. The algorithm gathers all this information and recommends products/services based on this behaviour.
How recommendation algorithms improve user experiences
A key benefit of recommendation algorithms is personalisation. By analysing data and user behaviour, algorithms can better understand preferences and therefore suggest more refined content or products. By personalising content, brands can offer a more intimate experience for their user. The net result of which increases trust and familiarity between the user and the brand.
Personalising a user journey also has the secondary benefit of increasing engagement. By offering them something that they are more likely to purchase they are more likely to spend longer on your site looking at other similar products.
Learning user behaviour and recommending relevant products increases the likelihood of them purchasing from your brand. This isn’t a hunch, either, this is backed by up a study from Bariliance. They found that personalised product recommendations saw a massive 70% increase in add-to-cart rates
Recommendation algorithms also save users time. By putting products they want to purchase front and centre they’re not wasting time (and losing interest) searching for the right product. Understanding behaviours and preferences algorithms helps reduce the ‘messy middle’ time between interest and purchase.
The experience a customer has on your brand’s website matters more than ever. A good experience means they are more likely to recommend you, leave reviews, and talk positively about your brand to friends and family. A great way to ensure they have a positive experience is by giving them the impression that you know what they want to buy. This can be done via a recommendation algorithm.
By providing a good customer experience, you also help to foster strong customer loyalty. Making their purchase journey easy by recommending products is likely to increase their fealty to your brand over a competitor.
The final notable advantage of using recommendation algorithms is the data and insights they provide. Understanding relevant audience behaviour means you can prioritise which products and services to promote to what audience segments.
Want to serve your audience better? Speak to our team today
Content first and recommendation algorithms are spearheading the way audiences interact with brands and find products.
So in order to be found by those with high buying intent audiences you need to be creating content that can leverage the benefits of recommendation algorithms.
Small to medium-sized businesses looking to make it in their industry no longer need to rely on building a mass following, all it takes is consistent, high-quality content.
To discover more about both our award-winning campaigns and how we can help leverage the benefits of recommendation algorithms get in touch today.